Institute News
Data science students explore the ethical considerations of their craft
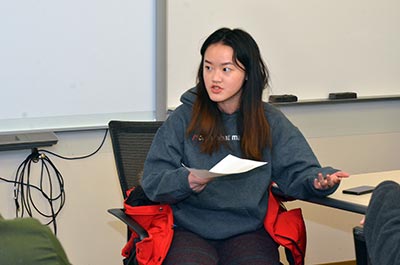
Data science makes it easier for police to monitor the activities of ordinary citizens, and for human resource departments to automatically whittle down a pile of job applications. Data science even enables Uber drivers to share among themselves who they consider to be “good” and “bad” customers.
However, as data science students are learning at the University of Rochester, all of these practices raise disturbing ethical questions.
“And it’s going to be up to your generation to help resolve these very important issues,” says Bob Berkman, who along with data and science outreach librarian Adrienne Canino helped create and teach a two-session module on ethics to 26 undergraduate and master’s students.
The capstone class, required of data science majors at the Goergen Institute for Data Science, matches teams of students with outside companies, who sponsor student projects to help address their data science needs. The class is taught by Ajay Anand, deputy director and instructor at the Institute.
“Given how data science is poised to impact society in many different ways now and in the future, incorporating teaching modules on ethics is an essential part of any modern data science curriculum,” Anand says. “As students in the course dive into different aspects of their data science project—data collection, pre-processing and building predictive models — the module encourages them to incorporate ethics into their decision making. Over time, we plan to offer dedicated courses in data science ethics keeping with the trend at universities nationwide.”
Berkman, an outreach business librarian with a special interest in data ethics, started the module with an example that made headlines in 2012—when Target, the retail chain, used predictive analytics to learn a teenage girl was pregnant before her own father knew. How? By correlating her shopping habits with other women who were expecting and then determining that there was a high probability that she, indeed, was pregnant.
“This has been written up as one of the classic case studies of the unanticipated consequences that can occur when algorithms and predictive analytics make assumptions about people,” Berkman said. “Often these assumptions are correct – not always – but even when they are right, they can be problematic.”
Uber drivers, for example, might understandably want to know ahead of time if someone asking for a ride late at night has been rated by other drivers as being unruly or argumentative. But could racial bias, for example, also enter the ratings, making it harder for certain groups to get the transportation they need?
After reviewing professional codes of ethics of the Association for Computing Machinery (ACM) and the Data Science Association (DSA), students were encouraged to offer their own opinions as Berkman and Canino introduced a series of other data-science scenarios and case studies. These included:
- A BBC video describing China’s camera surveillance network, one of the most advanced in the world, which uses facial recognition software to monitor peoples’ movements.
- Facebook’s’ controversial mood experiment, in which 700,000 users were unwittingly subjected to primarily positive or negative newsfeeds.
- The use of social networks as a platform for banks to judge credit worthiness.
On the second day, students split into teams. Each team was assigned to argue either the pros or the cons of using data science in an ethical “grey” area.
For example, an HR department in a large financial company wants to develop an algorithm that correlates job applicants’ consumer credit scores with the likelihood of longevity in a job, so it could then hire accordingly. This would help the company address an ongoing problem: New hires who move within a year or two to other companies.
This prompted a lively exchange.
The team assigned to discuss the benefits of this approach admitted to being initially concerned about the ethics of this approach. But they conceded that it “seems like a pretty reasonable practice” – as long as applicants consent to supplying the scores, the information is kept secure, and a correlation is actually established linking good scores to longevity.
The team assigned to discuss the unethical aspects of this approach drew upon the codes of ethics discussed earlier in the module.
For example, examining credit scores, in addition to the other traditional qualification applicants provide, “violates the principal of using minimal data necessary,” one team member said. “A credit score gives you a lot more than you need.”
A major concern was that use of credit scores would introduce socio-economic biases that would discriminate against some groups unfairly.
“If you see people are late paying of their credit balance, it could create an assumption that they are disorganized, and may not be the best workers. But it could be because of a whole bunch of things that we just don’t know.”
Thus, the practice would risk violating a DSA code of ethics rule against “misuse of weak or uncertain evidence to communicate a false reality or promote an illusion of understanding.”
(Berkman and Canino plan to continue to extend the library’s willingness and ability to share knowledge, trends, and instruction in data ethics with interested parts of the University of Rochester community.)
Student Comment on the Module
Joe Buckley
Master’s student in data science
I got a lot of insight from discussing these topics with my classmates. It was great to hear firsthand the viewpoints of students from China who grew up in a more regulated society in contrast with American ideals of liberty, and to better understand the trade-off between safety and security versus maintaining privacy rights. After our discussion, I felt that I understood more why someone would want to implement a state system that, for example, uses facial recognition to identify criminals, because of the order it upholds and the sense of safety and well-being it brings to law-abiding citizens.
Overall, the module was very helpful. Even better would be if the module could be expanded into a 4-credit class, perhaps jointly taught by a data science industry expert and a philosophy professor, and become standard in the major, in the same way bioethics classes are taught for hopeful physicians.
Fawzi Ali
Master’s student in data science
The ethics module was great to see in a typically technical-heavy course program. While going through real-life examples of ethics violations, it became apparent that there are many ways to avoid bias when collecting and analyzing user data. It's important to note that the implications of violating rules of ethics in data science may not be immediately obvious. It takes time to recognize implicit bias when conducting a project that takes advantage of user data.
Yankun Gao
Master’s student in data science
The module was very helpful. In our other classes we have concentrated on the knowledge or techniques that allow us to dig as much information as we can from the data. But we rarely thought about how to use the data properly in an ethical way. We want to collect useful information, and benefit people and make people's lives more convenient eventually. We do not want to hurt anyone. In the future, I will always keep this in mind in my work and use data properly.